ARtificial inTelligence for Integrated ICT-enabled pharmaceUticaL mAnufactuRing
When making new medicines it is very difficult to avoid "starting at the beginning" for every new drug that needs to be manufactured, which is very costly with new medicines currently doubling in cost every nine years; $1 billion US Dollars currently "buys" only half a new drug so addressing this issue is key for sustainability of the industry and future medicines supply.
ARTICULAR sought to develop novel machine learning approaches, a branch of artificial intelligence research, to learn from past and present manufacturing data and create new knowledge that aids in crucial manufacturing decisions. As a collaboration between the Glasgow School of Art, Loughborough University and the University of Strathclyde, it developed novel AI approaches for pharmaceutical manufacturing. The project was funded by a £2 million EPSRC Digital Manufacturing Award and supported by a £300k in-kind contribution from the below partners. Machine learning from development and manufacturing data and models were used to address common pharmaceutical product development problems.
Augmented Reality (AR) demonstrator for medicines manufacturing
Glasgow School of Art developed a number of XR tools for use in medicine manufacturing within the ARTICULAR project. Specifically, tools for facilitating the disassembly and reassembly of complex lab equipment, XR tools for lab design and equipment familiarity training.
Our team of 3D modellers and programmers accurately created digital replicas of various items of laboratory equipment providing the base ingredients for digital twins including filters, MSMPR, filter Dryer and twin-screw extruders. These models contained base operative functionality enabling users to interact with the equipment in XR environments for training purposes.
Through carefully designed experiments, we have proven the benefits of XR for training and teaching compared to traditional training methods.
CMAC AI-Enhanced Solubility App
A unified Machine Learning (ML) framework for solubility prediction across organic solvents
Used a single ML-based model to predict the solubility of drug/drug-like compounds across 49 organic solvents, extensible to more
By adopting a cross-solvent data structure, we enabled the exploitation of valuable relational information between systems
The effect was major, with even a single experimental measurement of a solute in a different solvent being enough to significantly improve predictions on it.
Robust Model-Based Reinforcement Learning for Controlling Crystallisation Processes
Advanced tracking control strategies for crystallisation using Reinforcement Learning.
Robust AI-based Control strategies of crystal size and shape disruptions under uncertainties and noisy data.
New control strategies that combined state-of-the-art MPC and AI learning capabilities.
Plug-and-play control strategies with Transfer Learning capabilities instead of single use control designs / tuning
Machine learning workflow to predict mechanical properties
Topological analysis-based persistence homology is used to represent crystal structures.
Computational calculation of elastic constants and mechanical properties.
Persistence barcodes and calculated elastic constants were used to develop machine learning models to predict mechanical properties of small organic compounds.
Papers
Benyahia, B.; Anandan, P. D.; Rielly, C., Control of Batch and Continuous Crystallization Processes using Reinforcement Learning, Computer Aided Chemical Engineering, 2021.
Ottoboni, S.; Wareham, B.; Vassileiou, A.; Robertson, M.; Brown, C. J.; Johnston, B.; Price, C. J., A Novel Integrated Workflow for Isolation Solvent Selection Using Prediction and Modeling. Organic Process Research & Development
Connor, L. E.; Vassileiou, A. D.; Halbert; G. W., Johnston; B. F.; Oswald, I. D. H., Structural investigation and compression of a co-crystal of indomethacin and saccharin. CrystEngComm 2019.
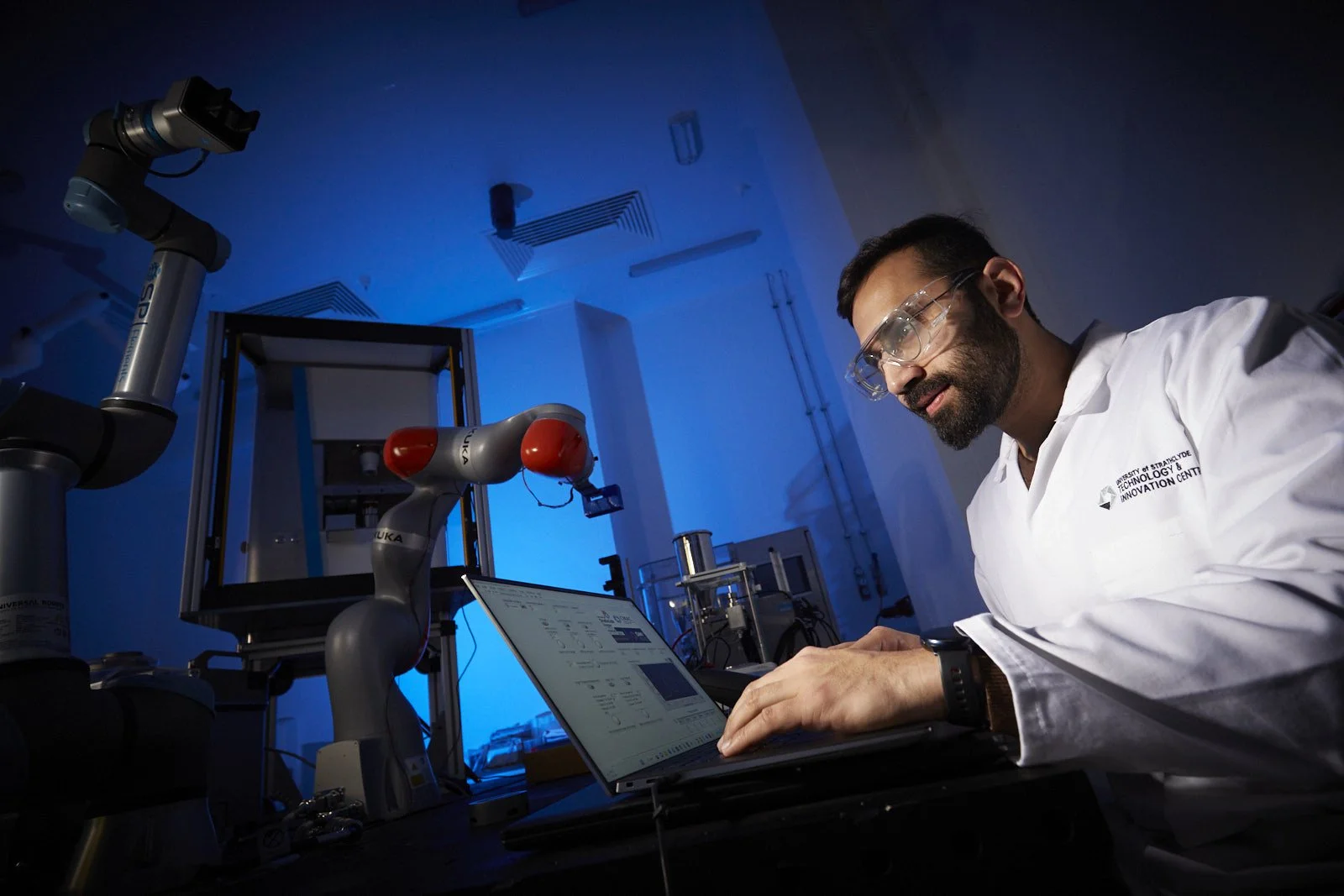

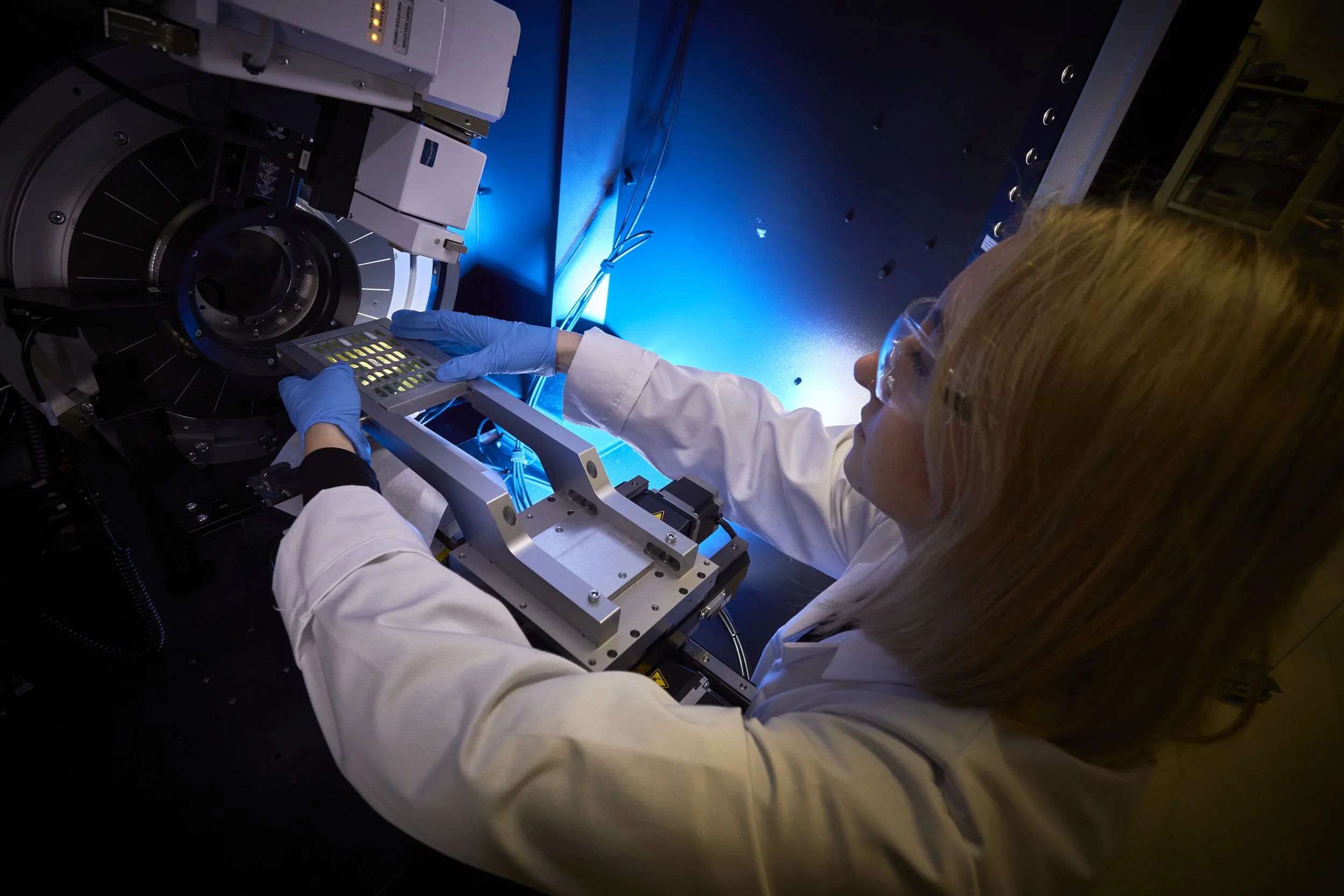


